by Karl Wirth, Macalester College,
Ed Nuhfer, California State Universities (retired)
Christopher Cogan, Memorial University
McKensie Kay Phillips, University of Wyoming
Introduction
Knowledge surveys (KSs) present challenges like exam questions or assignments, but respondents do not answer these. Instead, they express their felt ability to address the challenges with present knowledge. Knowledge surveys focus on self-assessment, which is a special kind of metacognition.
Overall, metacognition is a self-imposed internal dialogue that is a distinguishing feature of “expert learners” regardless of the discipline (e.g., Ertmer & Newby, 1996). Because all students do not begin college as equally aware and capable of thinking about their learning, instructors must direct students to keep them in constant contact with their metacognition. Paul Pintrich, a pioneer in metacognition, stressed that “instruction about metacognition must be explicit.” Knowledge surveys enable what Ertmer & Newby and Pintrich advocate in any class in any subject.
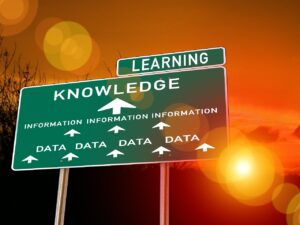
Knowledge surveys began in 1992 during a conversation about annual reviews between the guest editor and a faculty member who stated: “They never ask about what I teach.” Upon hearing the faculty member, the guest editor decided to create a 200-item form to survey student ratings of their mastery of detailed content for his geology course at the start and end of the class. The items were simply an array of test and quiz questions, ordered in the sequence the students would encounter during the course. The students responded to each item through a 3-point response at the start and end of the course.
The information from this first knowledge survey proved so valuable that the guest editor described this in 1996 in a geology journal as a formative assessment. As a result, geoscience faculty elsewhere started taking the lead in researching them and describing more benefits.
In 2003, U.S. Air Force Academy’s physics professor Delores Knipp and the guest editor published the first peer-reviewed paper (Nuhfer and Knipp, 2003) for multiple disciplines. If new to knowledge surveys, click the hotlink to that paper now and read at least the first page to gain a conceptual understanding of the instrument.
Self-assessment, Metacognition, and Knowledge Surveys
Becoming educated is a process of understanding self and the phenomena that one experiences. Knowledge surveys structure practices in understanding both.
Our series’ earlier entries revealed the measurable influence of self-assessment on dispositions such as self-efficacy, mindset, and intellectual and ethical development that prove indispensable to the lifelong process of becoming educated. The entries on bias and privilege revealed that the privilege of having the kind of education that renders the unconscious conscious may determine the collective quality of a society and how well we treat one another within it.
Knowledge surveys prompt self-assessment reflections during learning every aspect of the content. Over a baccalaureate education, cumulative, repetitive practice can significantly improve understanding of one’s present knowledge and self-assessing accuracy.
Improving Learning
Knowledge surveys’ original purpose was to improve student learning (e.g., Nuhfer & Knipp, 2003; Wirth et al., 2016, 2021). Providing students with a knowledge survey at the beginning of a course or unit of instruction offered an interactive roadmap for an entire course that overtly disclosed the instructor’s intentions for learning to students.
Early on, users recognized that knowledge surveys might offer a measure of changes in learning produced by a unit of instruction. Demonstrating the validity of such self-assessed competence measures was crucial but was finally achieved in 2016 and 2017.
Deeper Reading
Students quickly learned the value of prioritizing knowledge through engaging with the knowledge survey prior to and during engaging in reading. The structure of the KSs enabled reading with the purpose of illuminating known learning objectives. The structure also primed students to understand concepts by using the reading to clarify the connectedness between knowledge survey items.
Rather than just sitting down to “complete a reading,” students began reading assignments with appropriate goals and strategies; a characteristic of “expert readers” (Paris et al., 1996). When they encountered difficult concepts, they displayed increasing effort to improve their understanding of the topics identified as being essential to understand the concept. Further, knowledge surveys facilitated mentoring. When students did not understand the material, they proved more likely to follow up with a colleague or instructor to complete their understanding.
Facilitating Acquiring Self-Regulation
Well-constructed knowledge surveys are detailed products of instructor planning and thinking. They communicate instructor priorities and coordinate the entire class to focus on specific material in unison. That students’ comments expressing they “didn’t know that would be on the exam” nearly disappeared from classroom conversations cannot be overly appreciated.
Replacing scattered class-wide guessing of what to study allowed a collective focus on “How will we learn this material?” That reframing led to adopting learning strategies that expert learners employ when they have achieved self-regulation. Students increasingly consulted with each other or the instructor when they sensed or realized their current response to a knowledge survey item was probably inadequate.
Levels and Degrees of Understanding
In preparing a knowledge survey for a course, the instructor carefully writes each survey item and learning objective so that learning addresses the desired mastery at the intended Bloom level (Krathwohl, 2002). Providing awareness of Bloom levels to students and reinforcing this throughout a course clarifies student awareness of the deep understanding required to teach the content at the required Bloom level to another person. Whereas it may be sufficient to remember or comprehend some content, demonstrating higher cognitive processes by having to explain to another how to apply, synthesize or evaluate central concepts and content of a course feels different because it is different.
Knowledge surveys can address all Bloom levels and provide the practices needed to enable the paired understanding of knowing and “feeling of knowing” like no other instrument. Including the higher Bloom levels, combined with the explicitly stated advanced degree of understanding as the level of “teaching” or “explaining” to others, builds self-assessment skills and fosters the development of well–justified self-confidence. A student with such awareness can better focus efforts on extending the knowledge in which they recognize their weakness.
Building Skills with Feedback
The blog entries by Fleisher et al. in this series stressed the value of feedback in developing healthy self-assessments. Knowledge survey items that address the same learning outcomes as quizzes, exams, assignments, and projects promote instructional alignment. Such alignment allows explicit feedback from the demonstrated competence measures to calibrate the accuracy of self-assessments of understanding. Over time, knowledge surveys confer awareness that appropriate feedback builds both content mastery and better self-assessment skills.
A robust implementation directs students to complete the relevant portions of a knowledge survey after studying for an exam but before taking it. After the teacher grades the exams, students receive their self-assessed (knowledge survey score) and demonstrated (graded exam score) competence in a single package. From this information, the instructor can direct students to compare their two scores and to receive mentoring from the instructor when there is a large discrepancy (>10 points) between the two scores.
Generally, a significant discrepancy from a single knowledge survey-exam pair comparison is not as meaningful as longer-term trends illuminated by cumulative data. Instructors who use KSs skillfully mentor students to become familiar with their trends and tendencies. When student knowledge survey responses consistently over- or under-estimate their mastery of the content, the paired data reveal this tendency to the student and instructor and open the opportunity for conversations about the student’s habitually favored learning strategies.
A variant implementation adds an easy opportunity for self-assessment feedback. Here, instructors assign students to estimate their score on an assignment or exam at the start of engaging the project and after completing the test or assignment prior to submission. These paired pre-post self-assessments help students to focus on their feelings of knowing and to further adjust toward greater self-assessment accuracy.
Takeaways
Knowledge surveys are unique in their utility for supporting student mastery of disciplinary knowledge, developing their affect toward accurate feelings of knowing, and improving their skills as expert learners. Extensive data show that instructors’ skillful construction of knowledge surveys as part of class design elicits deeper thinking and produces higher quality classes. After construction, class use facilitates mutual monitoring of progress and success by students and instructors. In addition to supporting student learning of disciplinary content, knowledge surveys keep students in constant contact with their metacognition and develop their capacity for lifelong learning.
In Part 2, we follow from our more recent investigations on (1) more robust knowledge survey design, (2) learning about temporal qualities of becoming educated, (3) student authoring of knowledge surveys, and (4) mentoring students with large mis-calibrations in self-assessed competence toward greater self-assessment accuracy.